SmartDelta shares key insights on anomaly detection using runtime monitoring data
What steps should you take when software passes testing but behaves unexpectedly during runtime? Several partners in the ITEA SmartDelta project and beyond are already a step ahead. They use the hidden gem of runtime monitoring data to observe and analyse their system behaviour during execution, ensuring that the system operates as expected.
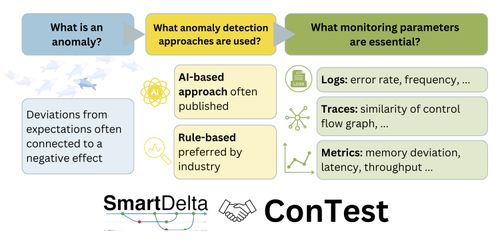
An international collaboration between the SmartDelta and ConTest projects was initiated to gather and synthesise information about the current state of anomaly detection from an industrial standpoint. This initiative involved discussions with industry partners and a systematic literature review on innovative anomaly detection techniques applied to real-world industrial datasets.
Key insights
Considering this collaboration, the following key insights were gathered:
- Most partners defined an anomaly as a deviation from expectations, where quick resolution is crucial to minimise negative impacts, aligning with standard IEEE definitions (ISO/IEC/IEEE 24765:2017). Summarising different project partners’ anomalies showed that anomalies heavily vary, making it difficult for anomaly detection algorithms to perform sufficiently.
- Extensive research has focused on AI-based approaches, while industry partners continue to favour rule-based approaches. Nonetheless, both approaches significantly rely on the availability of high-quality datasets for their effectiveness.
- But what are the essential parameters in their runtime monitoring data? Three types of monitoring data were identified: logs, traces, and metrics (as illustrated in the figure), along with their respective parameters for successful anomaly detection.
Rethinking the input parameters for anomaly detection
Enhancing the quality of the collected datasets and refining detection approaches by focusing on our detected key monitoring parameters, understanding interrelationships, and their impact on the system or microservices is essential. By incorporating the identified key input parameters, companies can enhance their approaches to detect a broader range of anomalies more quickly and reliably. Future work will establish a statistical model based on these parameters that provides insights into the parameters’ relationship and necessity.
Further insights
To learn more about this collaborative initiative of the SmartDelta and ConTest projects, have a look at their 2024 SEAA conference paper. Its current preprint is provided at: https://arxiv.org/abs/2408.07816
More information
Related projects
SmartDelta
Automated Quality Assurance and Optimization in Incremental Industrial Software Systems Development