A Deep Learning project – PAPUD’s lessons learned
Today, companies are generating huge amounts of data through their activities. Without an adapted analysis, these large amounts of heterogenous data go to waste.
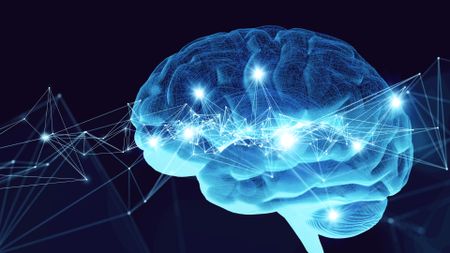
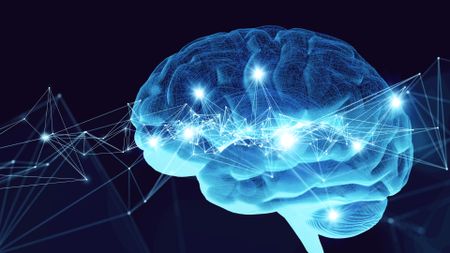
The aim of the ITEA project PAPUD is to help companies to exploit their large amounts of heterogenous data. Within a platform based around a shared architecture, the project has developed models and algorithms for data analysis with a particular focus on Deep Learning. By tailoring the analyses of both structured and unstructured data to different domains, PAPUD provides a competitive advantage for companies in the targeted markets.
The project outcomes proved the efficiency of the Deep Learning techniques applied to the following fields: e-commerce, call centre operation, human resources, e-government and HPC prescriptive maintenance. In a recent publication by PAPUD project leader Cornel Crisan from Bull Atos Technologies, some of the key lessons learned are shared. The experiences of the PAPUD project are exchanged including valuable insights about areas such as hardware platforms, infrastructure, libraries commonalities, inference through web applications and the experience of a Deep Learning collaborative project.
The story is a message to industry as a whole: it is becoming significantly easier for companies to benefit from data analytics. Use of the PAPUD platform and the creation of domain-specific Deep Learning tools allow businesses to bypass huge organisations which have dominated the field, saving them time and money while also allowing them to tailor resources more specifically to their own internal or commercial needs. This should then provide a competitive advantage over companies which fail to make the most of Deep Learning. You are encouraged to read the full article to integrate or evaluate the Deep Learning techniques for all kind of projects, especially for research projects using or generating large amounts of data.